At The Hyve we want to empower scientists by building upon open source software. With tools like TranSMART we store data and give scientists the opportunity to analyse and browse their data. However, these functionalities are only possible inside TranSMART. How amazing would it be, if you could find any dataset you want and make it work with your own dataset?
We at The Hyve noticed during the years how much data is lost during research, data which can be used by other scientists to excel their own research. Due to the recent developments surrounding FAIR data, we have decided to research how we can make our own open source tools FAIR. We asked ourselves: "What needs to be done and how FAIR can we go already?"
FAIR data?
The goal of FAIR data is to make data: Findable, Accessible, Interoperable and Re-usable. By describing data with rich metadata, a machine can interpret the data and “know” what the dataset contains. The machine also has the possibility to convert this metadata to a human readable format, or visa versa. With the machine knowing what is inside the data, it can make the data interoperable with other datasets.
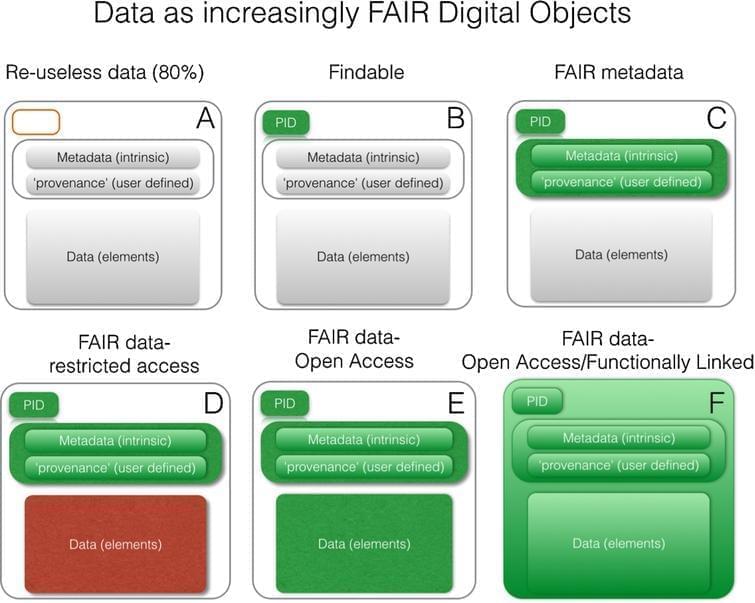
2016 was a big year for FAIR data. It started with the publication of the FAIR Guiding Principles in Nature Scientific Data. Although the principles seem abstract, they clearly describe what is needed to make a tool FAIR. While reading through the principles, it became clear that advancing a tool to become FAIR will take a significant amount of work.
With the recent follow-up paper stating degrees of FAIRness, the route to a FAIR world became a lot more clear. There is no FAIR and not FAIR, rather it is a gradient. By complying to the principles for Findable and Accessible you can achieve certain levels of FAIRness, without it being a big investment in resources.
Meanwhile, our partner DTL has started with creating technical implementations of the FAIR principles. This showed us, that a lot of the technology needed for FAIR data is already present. With this in our minds, we decided to take one of our main open source tools on a test drive in the world of FAIR data.
Taking TranSMART on a test drive
Our first significant step in the FAIR data world was a hackathon for TranSMART, which took place on the 16th of February. By comparing TranSMART with the FAIR principles, we checked which principles were already met and which were not. There upon we looked at how we could implement the missing principles. During this phase we decided that the focus should be on Findable and Accessible in the first place. After discussing with the team how we could achieve this, we concluded that the following two additions will significantly raise the level of FAIRness of TranSMART:
- A way to add FAIR metadata to your study as tags in TranSMART.
- A FAIR Data Endpoint. This is an API, that gives you the possibility to hook up your TranSMART to a FAIR search engine. This search engine can be connected with other TranSMARTs around the world and with other tools that have already achieved this level of FAIRness. So when your TranSMART is connected with the search engine, you could exchange studies from your TranSMART with other TranSMART instances. Or visa versa import studies from other TranSMART instances.
The Future
Making TranSMART fully comply to the FAIR Guiding Principles will take a significant amount of resources. Nonetheless raising the level of FAIRness of TranSMART is definitely worthwhile, compared to the benefits it will give to the community. And the good news? We already started with developing the FAIR data point and the additions needed to the Arborist. In the coming weeks we will release a white paper on how we are going to achieve this.
Are you just as excited about FAIR data as we are? Contact us to see how we can help you make your data FAIR.
Reference image: Cloudy, increasingly FAIR; revisiting the FAIR Data guiding principles for the European Open Science Cloud