Combining data from different study types can lead to interesting new insights. Imagine your organization wanted to combine clinical trial data with omics data to investigate any relationships in their response to specific treatments. To enable this, their data had to become more Findable, Accessible, Interoperable, and Reusable (FAIR).
In this situation, a data landscape becomes essential. But how can you create a data landscape that aligns with FAIR? Let’s delve into what a data landscape is.
Understanding what is Data Landscaping:
Data landscaping involves meticulously mapping and organizing existing data sources within an organization. It provides a panoramic view of an organization’s data assets, data sources, storage options, and the systems involved in creating, analyzing, processing, and storing data that can be scoped to specific business areas.
Data landscaping can also be domain-specific, considering how data exists, evolves, and interacts within specific domains. This perspective involves assessing the current state (how data is generated, received, processed, and used) and the future state (aspirations for data management, including improvements, innovations, and strategic goals).
For example, in clinical trials within a pharma organization, researchers can examine their current state of data collection during trials, how it is reported, and how it is used with other sites. The overall aim is to streamline this data capture process to improve trial efficiency.
When it comes to the life sciences industry, the data in question is research data, clinical trial data, real-world healthcare data, and more. Expert knowledge of scientific domains, data standards, and computational frameworks is needed for a data landscape exploration in these domains.
Opportunities arising when Data Landscaping exploration is needed:
FAIRify data: To enhance its reusability, thus maximizing its value.
Complex Data Integration: Simplifying the consolidation of diverse data sources for comprehensive analysis.
Data Quality Assurance: Ensuring data accuracy and consistency for reliable insights.
Disorganized Workflow: Incorporating exploratory data analysis into workflows for better decision-making.
Reduce Operational Costs: Streamlining data management processes leads to operational efficiencies, cutting down on time and resources spent on data-related tasks.
With that in mind, Data Landscaping exploration can be applied at each stage of a researcher’s workflow process, as depicted in Figure 1 below.
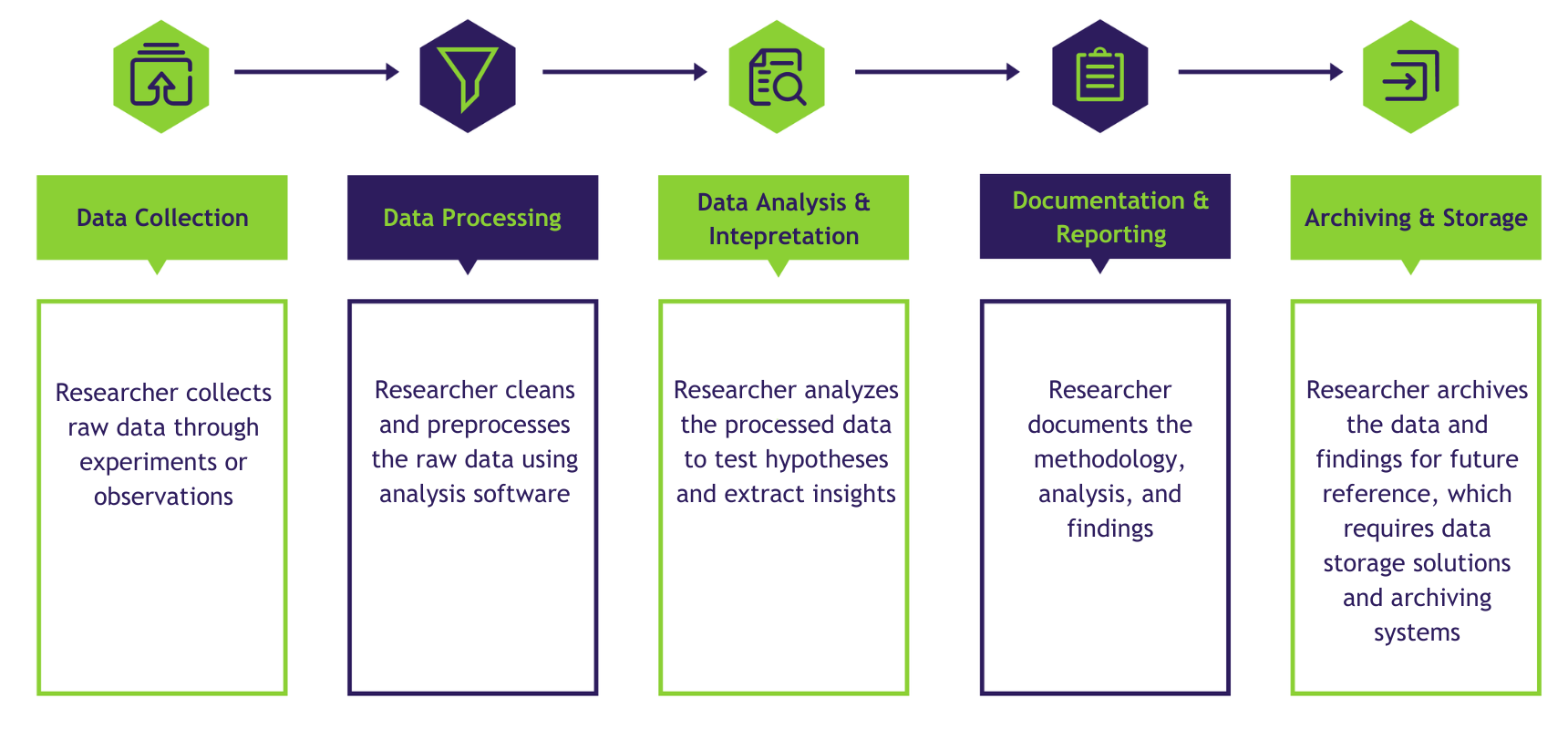
Value of Data Landscaping
Let’s explore the value:
Create and improve Data strategy- We provide organizations with an in-depth understanding of their current data landscape. This facilitates data management and information requests, enabling an informed exploration of data management needs and data flow across various departments and domains. This allows organizations to make informed decision-making and strategic planning when it comes to their overall data management and investments in technology/tools to save costs.
Optimizes Research Spending - By identifying the most valuable data sources and eliminating redundant data collection, funding can be directed to where it’s most impactful.
Advancing AI and Hypothesis Readiness - In the rapidly evolving technological landscape, it’s crucial to stay ahead. Our data landscaping services ensure that data is not only AI-ready but also primed for hypothesis testing. This proactively positions organizations steps ahead of competitors who are swiftly adopting AI and machine learning models, thus accelerating their research and development workflows.
In principle, Data landscaping aligns with the principles of FAIR data, and this is where The Hyve can ensure this happens at the required stages:
Findable: By mapping data sources, we make them discoverable.
Accessible: Organized data becomes accessible to researchers across the organization.
Interoperable: A well-structured landscape facilitates seamless data exchange.
Reusable: Reusable data accelerates scientific progress. A well-landscaped data environment ensures that data can be reused effectively. Researchers can harvest insights from existing datasets, avoiding redundant efforts and fostering collaboration.
The Hyve approach to FAIR By Design Data Landscaping
The Hyve, with its expertise in bioinformatics, FAIR, and life sciences, has helped pharma, academia, and research institutions in a FAIR by Design data landscape. The Hyve team can tailor a data landscape activity to different types of outcomes/deliverables:
- FAIR-by-Design Data Strategy:
We collaborate with your team to craft a robust data management strategy.
Our focus: making data FAIR (Findable, Accessible, Interoperable, and Reusable), at every stage of its lifecycle.
- Recommendations for FAIR-by-Design Architecture:
Our team evaluates your existing data infrastructure.
We provide tailored recommendations for designing a FAIR-by-design architecture or the tools that are according to your specific needs and use cases.
- Semantic modeling to guide researchers in uncovering insights easily:
Our team of data modelers can derive specific domain models after a data landscape activity.
Creation of conceptual or/and semantic models that can be implemented inn your data platform.
Figure 2 below is an outline example of a typical project approach for an organization seeking a fair-by-design data strategy. This approach aims to create a comprehensive report that can be presented to their board of directors.
Figure 3 below is an outline example of a typical project approach for an organization seeking a fair-by-design data architecture. This approach aims to create a comprehensive report designing the type of architecture that would suit the organization’s objectives or recommending the tools according to those objectives. This can be used to support technology decisions.
Integrating Large Language Models into Your Data Landscape
Integrating Large Language Models (LLMs) into your data landscape may enhance your business operations by making data more accessible, actionable and AI ready across the organization. Here at The Hyve, we specialize in building LLM solutions tailored to your requirements, and we will consider, for example, some of the following for an organization: How to help organizations make the best use of LLMS or How to use LLMs with knowledge graphs to make data AI ready.
When to start? Now!
Data landscapes are dynamic, and regular exploration is key to maintaining alignment with evolving business objectives and technological breakthroughs. In the realm of biomedical research, the true potential of data is unlocked when it is carefully managed, enriched, and collaboratively utilized. With the rapid advancement of technologies such as AI, there’s no time to pause; readiness is not an option but a necessity. By proactively preparing your data for these technologies, you can accelerate your research endeavors, yielding significant savings in time, costs, and resources, while preemptively addressing potential challenges in data management workflows.
Let’s embrace data landscaping as a vital tool in our scientific toolkit. Remember, a FAIR data landscape empowers life sciences organizations to optimize data utilization effectively.
For further information or to explore our FAIR by Design data landscaping services, please reach out to melissa@thehyve.nl or office@thehyve.nl.